The Future of Healthcare in the Age of AI - Perspectives from our work at CareCentra
By
David Kinney, Ph.D Lead Decision Scientist,
Vasant Kumar, Founder & CEO at CareCentra
.png)
Several studies have shown that clinical care, both access and quality, account for only 17 percent of variations in health outcomes. Socioeconomic factors alone may account for 47 percent, health behaviors 34 percent, and physical environment 3 percent of health outcomes, respectively.
1 Imagine the ability to collect a wide variety of risk markers and signals across a population and convert these into actionable intelligence in real time at the level of an individual patient! Further imagine, based on these insights, personal algorithms recommending ‘do this’, don’t do that’ like a personal caregiver might. These recommendations empower each individual to take greater charge of their own care by taking an optimal or near-optimal health action at any given time.
Until recently, it was impossible to collate longitudinal data across all these factors beyond clinical care (largely claims and clinical encounters data), to attempt any meaningful analysis for managing health risks. However, the era of AI-enabled personalized care is now upon us. Increasingly, algorithms can take on board whole health data at the patient-level – including social determinants of health, lifestyle behaviors, and environmental and clinical data – and output a care plan tailored to that patient’s risk, lifestyle, and socioeconomic context. Indeed, computational models now allow for a unified view of the patient’s health risks. Advances in LLMs can translate insights from these views into personalized lists of do’s and don’ts that eventually help mitigate those risks. However, like all AI methods, AI-supported personalized care is subject to the “garbage-in, garbage-out” problem. The patient data that an algorithm receives must be coarse-grained enough to be tractable and explainable, while still probative enough to generate a signal as to the kinds of strategies that will work especially well for a given patient.
Evidence from our work at CareCentra
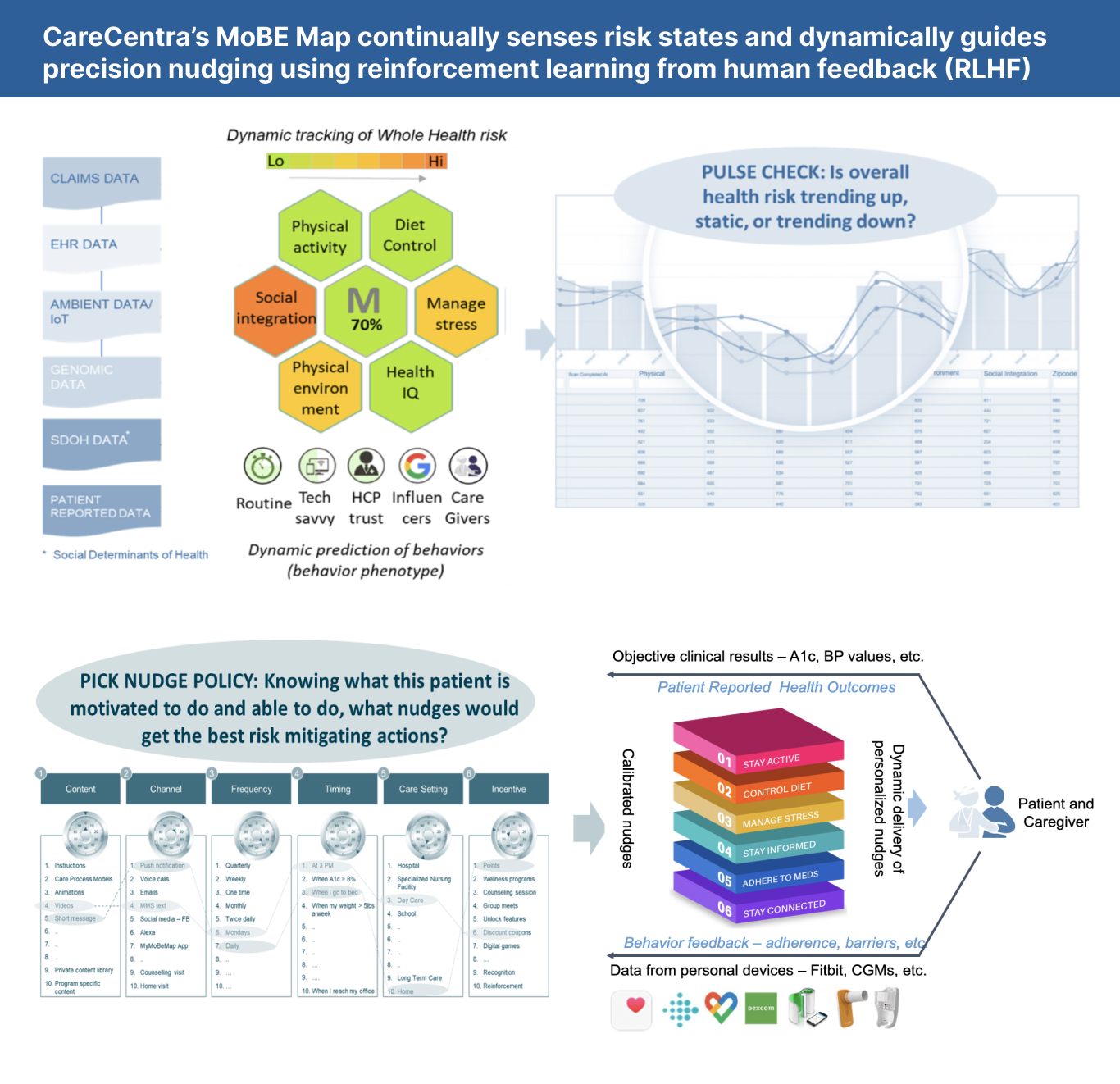
At CareCentra, we use a propriety method for phenotyping the factors that shape a patient’s motivation and abilities – the MoBe Map – to generate patient-specific insights that shape behaviors at an individual level while improving outcomes at scale. When patients are onboarded into a CareCentra program, they answer a survey that aims to categorize where that patient falls along several different motivational and ability axes (e.g., is the patient tech savvy, do they deal with stress well, do they have strong social ties, are they capable of maintaining a healthy diet, etc.). Upon completing these questions, they are assigned a ten-component “MoBe vector” representing their position along these different axes. This allows us to investigate the relationship between a patient’s MoBe vector and various health outcomes, and target interventions to an individual patient based on these findings. Moreover, statistical analysis allows us to drill down on the handful of factors that matter in each health context. For instance, in one cohort of patients, we found that three MoBe factors were associated with more positive outcomes: strength of social ties, ability to handle stress, and tech savvy. This knowledge enables us to design interventions that specifically target patients with lower scores on these factors.
Technical approach and methods
To arrive at this conclusion, we performed a LASSO (least absolute shrinkage and selection operator) regression and feature selection procedure that tested the ability of each MoBe factor to predict positive outcomes in real patients. A LASSO regression aims to learn a linear model that relates independent variables to dependent variables, while enforcing the requirement that the sum of the magnitudes of all coefficients be no greater than a regularization parameter. This requirement forces the regression coefficients for unimportant predictors to zero, leaving only the important predictors of the dependent variable – in this case, the length of a maternity patient’s pregnancy. After using cross-validation to find an optimal regularization parameter, we found that the three MoBe factors listed above – strength of social ties, ability to handle stress, and tech savvy – were positive predictors of pregnancy length, with higher values of each associated with better outcomes. This then enables us to fine-tune the output of generative AI applications, such as LLMs, to produce content aimed specifically at patients with lower scores in some combination of these three areas, all while keeping a human in the loop. These generated interventions can be coupled with our reinforcement learning algorithms to learn optimal, patient-specific policies in a wide range of health contexts: recent results have demonstrated the efficacy of this same approach in a medication adherence setting.
Why is this important to the future of healthcare?
Patients produce an enormous amount of data. To use that data to customize a treatment plan while still having a clear answer to the question “why was this treatment chosen for me?”, we need to deploy dimensionality-reduction and feature selection techniques on the data we have. Doing this allows us to isolate clinically important behavioral factors, which in turn enables clinicians and patients to work together to formulate a bespoke treatment plan for the patient, all with the goal of increasing patient flourishing at all stages of their life cycle.
Reference: