The Future of Healthcare in the Age of AI - Perspectives from our work at CareCentra
By David Kinney, Ph.D Lead Decision Scientist, Vasant Kumar, Founder & CEO at CareCentra
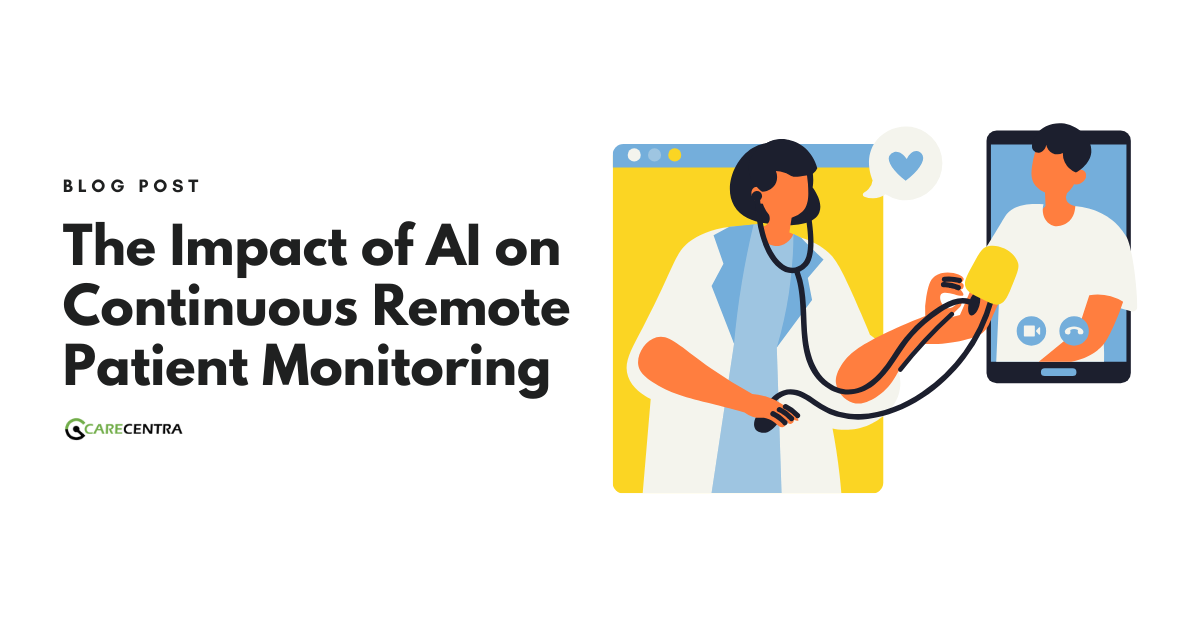
The application of AI models to data from wearables and remote health monitors is catalyzing precision healthcare. Algorithms can now assess patient risk without exclusive dependence on clinical data or PHI by using signals from a combination of wearables, patient reported outcomes, and data on social determinants to continuously monitor health risks, thus enabling early anomaly detection and prompt interventions. For example, algorithms detecting patient stress/anxiety levels with reasonable accuracy from wearables, can now suggest lifestyle interventions before any clinical escalation. While pinpoint accuracy is unrealistic, determining risk direction is often sufficient for prompting behavioral interventions. Importantly, once well-calibrated, these models enable such capabilities despite uneven data flows in time which is crucial for real-world patients with inconsistent schedules.
Evidence from our work at CareCentra
Integrating data from remote monitors and wearables, when appropriately processed, allows CareCentra’s AI to shape patient's health behaviors at scale. Our algorithm combines a wide variety of user-consented data, without mandating any of it. These include:
- Lifestyle behavior data - diet, exercise, stress, sleep, and adherence to medications (without the need for prescription names since the focus is on patient behavior)
- Social determinants from patient geographies (indexed for air quality, housing, health infrastructure, transportation, etc. from open-sourced data)
- Patient Reported Outcomes Measures and symptom checker data
- Mood and stress profiles and social integration metrics derived from survey data
Our neural net models perform ~20-24% better when patients integrate data from their wearables and remote IoT monitors to the App they interact with daily (MyMoBeMapTM). These same models process a combination of objective and subjective health indicators (e.g., both sleep statistics and mood check responses) to suggest non-clinical interventions that bend the risk curve in the case of hypertension patients on our cardiovascular care journey.
Technical approach and methods
Our proprietary, self-learning,
Unified Health Algorithm is powered by neural network models, including liquid neural nets and other convolutional neural nets. These models are trained on data from wearables and remote monitors, combined with other patient lifestyle and behavior data, to generate a behavior propensity profile for each patient (the MoBE Map) that informs risk predictions and driving precision nudges. We add a dense variational hidden layer to our neural network, and this allows us to use Bayesian inference and estimate a reasonable confidence interval around our predictions. Embracing this probabilistic approach is essential in a domain like medicine where both aleatory and epistemic uncertainty is inevitable. This enables us to sense actionable risk trends and apply adaptive nudging techniques to personalize action triggers for patients.
Why is this important to the future of healthcare?
The adoption of applied machine learning algorithms in healthcare, alongside increased deployment of wearables, is driving the transition from a model focused on episodic care to one focused on continuous, preventive care. Early detection of risk improves the patient's experience of care by shaping appropriate mitigatory responses through early self-care, often before exacerbations. One can think of these algorithms as the healthcare analog of the "check engine" light on the dashboard of a car, reducing administrative burdens on clinicians and staff by intelligently addressing warning signals early, and by promoting team-based care. Taken together, wearables data, deep learning techniques, and minimal clinician support as needed, amount to a symphony that can improve outcomes and reduce cost burdens throughout the healthcare system.